Diagnostic imaging remains an essential tool for the detection and treatment of many disorders. In numerous clinical settings, an X-ray often serves as the starting point for diagnosis. However, to deliver that diagnosis, a radiologist must identify and evaluate the X-ray. As a result, competition can be fierce for the radiologist when numerous imaging studies are in their queue. Artificial Intelligence (AI) is a powerful tool with the potential to alleviate the demand on radiologists by doing preliminary evaluations on medical images and organizing workflow to improve efficiency.
There is already promising evidence that AI can perform complex diagnoses. According to a 2020 study, AI can distinguish between four different diseases in chest x-rays: 1) pulmonary malignant neoplasms, which includes lung cancers and metastasis; 2) active pulmonary tuberculosis, 3) pneumothorax; and 4) pneumonia. AI’s ability to help radiologists identify patients with complex cases is growing and will continue to evolve to cover more diagnoses categories.
AI will be particularly impactful in helping radiologists address an increasing number of imaging scans they must read quickly, allowing more them to look at more scans in less time. Reducing the time required for reading images enables radiologists to have more time to analyze data from sources beyond image data and spend more time on direct patient care. Radiologists have training in quality control, management, performing minimally invasive procedures, and acting as consultants. They have the expertise and knowledge to select the most appropriate imaging modality to address a clinical issue. This extra time will position radiologists to provide input to others in the healthcare team to ensure patients have the most appropriate imaging scans performed, allowing best for detection of a diagnosis.
Despite the potential benefits of AI technology, a recent survey showed only 38% of radiologists had been exposed to one of the five most common use cases of AI in radiology today: 1) optimizing workflow for productivity, 2) tagging images so critical patients are the first reviewed, 3) automating part of the image analysis, 4) enhancing imaging quality, and 5) providing decision support [2]. AI in medical imaging can identify imaging abnormalities and improve tissue-based detection and characterization.
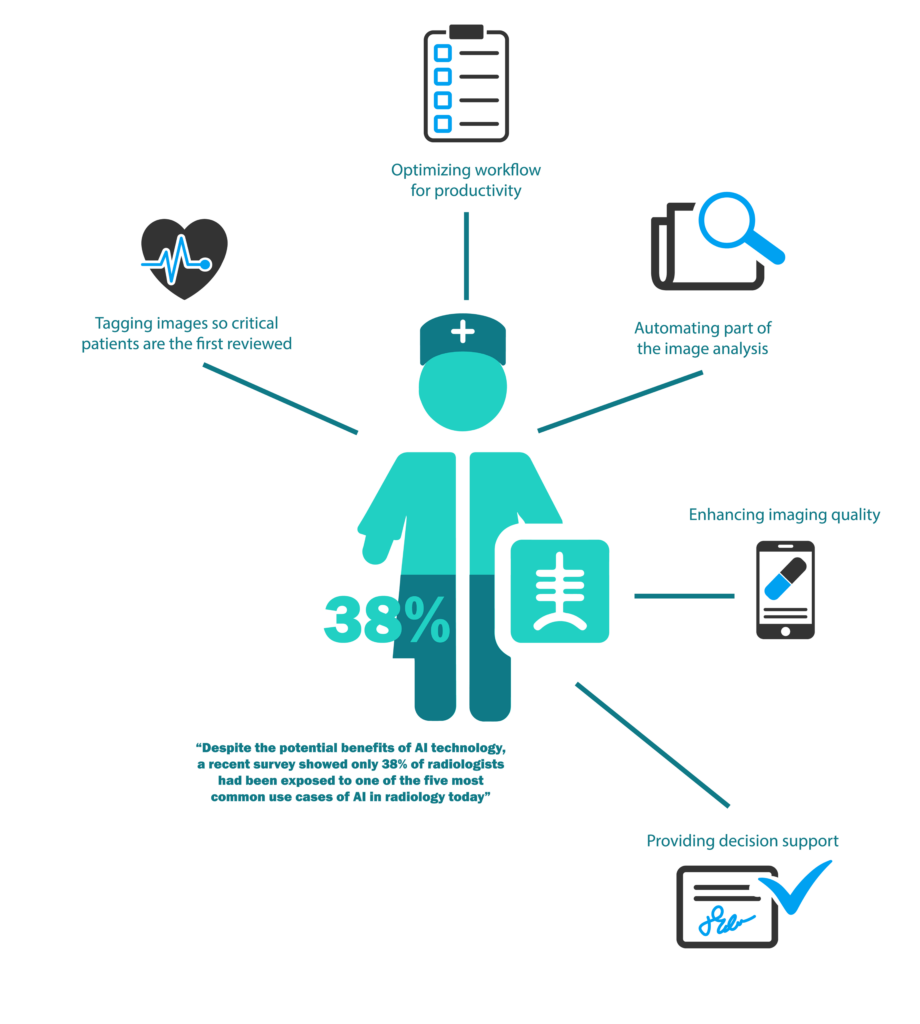
There are real challenges involved with the implementation of AI techniques in medical imaging. The human body has structures that have wide variations of typical dimensions and textures which can potentially mask pathological conditions. Additionally, diagnoses cannot always be agreed upon and proven with certainty. The ideas behind diagnoses evolve with updating classifications, concepts, and increasing knowledge.
The slow implementation of AI is a result of lack of trust in its capabilities. In a recent survey, 40% of respondents believe the biggest barrier to adopt AI in medical imaging is themselves. This reluctance is primarily driven by skepticism in AI’s current diagnostic capabilities, particularly for more complex patients or disease states. By positioning AI technology as a useful, supplemental tool, radiologists and other clinicians can reap the benefits of this technology as their confidence in the ability of AI grows.
The impact of AI should slowly become part of the routine of the radiologist. Radiologists will prioritize examinations based on disease severity and glean additional information from images not currently utilized. AI has already shown capable of generating parts of the radiological report, such as a preliminary description of the image’s findings and some measurements of lesions identified. These enhancements will allow the radiologist to integrate information with clinical data better and contribute holistically to the diagnoses and individualized treatment plans.
Ultimately, AI in medical imaging can lead to better outcomes for patients. Radiologists who embrace AI’s medical imaging transformation will help improve patient care and allow patients to have more confidence in a diagnosis. Be sure to stay tuned for Ellumen’s continuing blog series on the delivery of AI innovation in medical imaging.
Meet Ellumen’s AI Experts
Are you looking to utilize AI for your next technology or integrate AI into your current systems? Feel free to reach out to us.

Iyanuoluwa Odebode is Ellumen’s artificial intelligence/machine learning expert, supporting the Innovation Lab project by identifying and researching new & emerging technology. He also serves as an adjunct professor in cybersecurity at University of Maryland Baltimore County. Prior to his work at Ellumen, Iyanuoluwa built an algorithm for repurposing old drugs for new use using the DReiM methodology. He has been published in ResearchGate and IEEE for his research on machine learning. Iyanuoluwa completed his master’s in bioinformatics at Morgan State University and his Ph.D. in information systems (machine learning/AI) at University of Maryland Baltimore County.
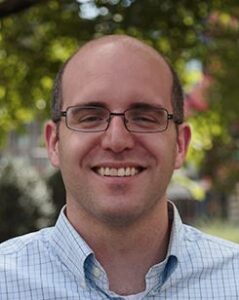
Todd R. McCollough is a Software Engineer for Ellumen. He works primarily with the CVIX/VIX Services which support image viewers and applications to provide the ability for users to query, retrieve and manipulate VA and DoD medical images and image artifacts. Todd is a co-inventor on several issued U.S. patents and is passionate about discovering novel ways to image patients and improve patient care. Todd received his bachelor’s and master’s degrees in biomedical engineering from Northwestern University.